Facial recognition is becoming part of the fabric of everyday life. You might already use it to log in to your phone or computer or authenticate payments with your bank. In China, where the technology is more common, your face can be used to buy fast food, or claim your allowance of toilet paper at a public restroom. And this is to say nothing of how law enforcement agencies around the world are experimenting with facial recognition as tool of mass surveillance.
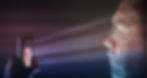
But the widespread uptake of this technology belies underlying structural problems, not least the issue of bias. By this, researchers mean that software used for facial identification, recognition, or analysis performs differently based on the age, gender, and ethnicity of the person it’s identifying.
A study published in February by researchers from MIT Media Lab found that facial recognition algorithms designed by IBM, Microsoft, and Face++ had error rates of up to 35 percent higher when detecting the gender of darker-skinned women compared to lighter-skinned men. In this way, bias in facial recognition threatens to reinforce the prejudices of society; disproportionately affecting women and minorities, potentially locking them out of the world’s digital infrastructure, or inflicting life-changing judgments on them.
That’s the bad news. The worse news is that companies don’t yet have a plan to fix this problem. Although individual firms are tackling bias in their own software, experts say there are no benchmarks that would allow the public to track improvement on an industry-wide scale. And so when firms do reduce bias in their algorithms (as Microsoft announced it had last month), it’s difficult to judge how meaningful this is.
Clare Garvie, an associate at Georgetown Law’s Center on Privacy & Technology, told The Verge that many believe it’s time to introduce industry-wide benchmarks for bias and accuracy: tests that measure how well algorithms perform on different demographics, like age, gender, and skin tone. “I think that would be incredibly beneficial,” says Garvie. “Particularly for companies who will be contracting with government agencies.”
WHAT ARE THE COMPANIES DOING?
In an informal survey, The Verge contacted a dozen different companies that sell facial identification, recognition, and analysis algorithms. All the firms that replied said they were aware of the issue of bias, and most said they were doing their best to reduce it in their own systems. But none would share detailed data on their work or reveal their own internal metrics. If you regularly use a facial recognition algorithm, wouldn’t you like to know whether it consistently performs worse for your gender or skin tone?
Google, which only sells algorithms that detect the presence of faces, not their identities, said: “We do test for bias and we’re constantly testing our underlying models in an effort to make them less biased and fairer. We don’t have any more detail to share around that at this time.”
Microsoft pointed to its recent improvements, including to its gender recognition software, which now has an error rate of 1.9 percent for darker-skinned women (down from 20.8 percent). The company didn’t offer official comment but pointed to a July blog post by its chief legal officer, Brad Smith. In the post, Smith said it was time for the US government to regulate its own use of facial recognition, though not its deployment by private firms. Including, perhaps, setting minimum accuracy standards.
IBM also highlighted recent improvements, as well as its release last month of a diverse dataset for training facial recognition systems, curated to combat bias. Ruchir Puri, chief architect of IBM Watson, told The Verge in June that the company was interested in helping establish accuracy benchmarks. “There should be matrixes through which many of these systems should be judged,” said Puri. “But that judging should be done by the community, and not by any particular player.”
Amazon also did not respond to questions but directed us to statements it issued earlier this year after being criticized by the ACLU for selling facial recognition to law enforcement. (The ACLU made similar criticisms today: it tested the company’s facial recognition software to identify pictures of Congress members and found that they incorrectly matched 28 individuals to criminal mugshots.)
Amazon says it will withdraw customers’ access to its algorithms if they are used to illegally discriminate or violate the public’s right to privacy but doesn’t mention any form of oversight. The company told The Verge it had teams working internally to test for and remove biases from its systems but would not share any further information. This is notable considering Amazon continues to sell its algorithms to law enforcement agencies.
Of the enterprise vendors The Verge approached, some did not offer a direct response at all, including FaceFirst, Gemalto, and NEC. Others, like Cognitec, a German firm which sells facial recognition algorithms to law enforcement and border agencies around the world, admitted that avoiding bias was hard without the right data.
“Databases that are available are often biased,” Cognitec’s marketing manager, Elke Oberg, told The Verge. “They might just be of white people because that’s whatever the provider had available as models.” Oberg says Cognitec does its best to train on diverse data but says market forces will weed out bad algorithms. “All the vendors are working on [this problem] because the public is aware of it,” she said. “And I think if you want to survive as a vendor you will definitely need to train your algorithm on highly diverse data.”
HOW CAN WE ADDRESS THE ISSUE OF BIAS?
These answers show that although there is awareness of the problem of bias, there’s no coordinated response. So, what to do? The solution most experts suggest is conceptually simple, but tricky to implement: create industry-wide tests for accuracy and bias.
The interesting thing is that a such test already exists, sort of. It’s called the FRVT (Face Recognition Vendor Test) and is administered by the National Institute of Standards and Technology, or NIST. It tests the accuracy of dozens of facial recognition systems in different scenarios, like matching a passport photo to a person standing at a border gate, or matching faces from CCTV footage to mugshots in a database. And it tests “demographic differentials” — how algorithms perform based on gender, age, and race.
However, the FRVT is entirely voluntary, and the organizations that submit their algorithms tend to be either enterprise vendors trying to sell their services to the federal government, or academics testing out new, experimental models. Smaller firms like NEC and Gemalto submit their algorithms, but none of the big commercial tech companies do.
Garvie suggests that rather than creating new tests for facial recognition accuracy, it might be a good idea to expand the reach of the FRVT. “NIST does a very admirable job in conducting these tests,” says Garvie. “[But] they also have limited resources. I suspect we would need legislation or federal funding support to increase the capacity of NIST to test other companies.” Another challenge is that the deep learning algorithms deployed by the likes of Amazon and Microsoft can’t be easily sent for analysis. They are huge pieces of constantly updating software; very different to older facial recognition systems, which can usually fit on a single thumb drive.
Speaking to The Verge, NIST’s biometric standards and testing lead Patrick Grother made it clear that the organization’s current role is not regulatory. “We don’t do regulation, we don’t do policy. We just produce numbers,” says Grother. NIST has been testing the accuracy of facial recognition algorithms for nearly 20 years and is currently preparing a report specifically addressing the topic of bias, due at the end of the year.
Grother says that although there have been “substantial reductions in errors” since NIST started tests, there are still large disparities between performance of different algorithms. “Not everyone can do facial recognition, but a lot of people think they can,” he says.
Grother says that recent discussion of bias frequently confuses different types of problems. He points out that although a lack of diversity in training datasets can create bias, so too can bad photography of the subject, especially if their skin tone is not properly exposed. Similarly, different types of errors mean more when applied to different types of tasks. All these subtleties would need to be considered for any benchmark or regulation.
BIAS ISN’T THE ONLY PROBLEM
But the discussion about bias invites other questions about society’s use of facial recognition. Why worry about the accuracy of these tools when the bigger problem is whether or not they’ll be used for government surveillance and the targeting of minorities?
Joy Buolamwini, an AI scientist who co-authored the MIT study on different accuracy rates in gender-identifying algorithms, told The Verge over email that fixing bias alone does not fully address these wider issues. “What good is it to develop facial analysis technology that is then weaponized?” says Buolamwini. “A more comprehensive approach that treats issues with facial analysis technology as a sociotechnical problem is needed. The technical considerations cannot be divorced from the social implications.”
Buolamwini and some others in the AI community are taking a proactive stance on these issues. Brian Brackeen, CEO of facial recognition vendor Kairos, recently announced that his company would not sell facial recognition systems to law enforcement at all because of the possibility for misuse.
Speaking to The Verge, Brackeen says that when it comes to commercial deployment of facial recognition, market forces would help eliminate biased algorithms. But, he says, when these tools are used by the government, the stakes are much higher. This is because federal agencies have access to much more data, increasing the possibility of these systems being used for suppressive surveillance. (It’s estimated that the US government holds facial data for half the country’s adult population.) Similarly, decisions made by the government using these algorithms will have a greater impact on individuals’ lives.
“The use case [for law enforcement] isn’t just a camera on the street; it’s body cameras, mugshots, line-ups,” says Brackeen. If bias is a factor in these scenarios, he says, then “you have a greater opportunity for a person of color to be falsely accused of a crime.”
Discussion about bias, then, looks like it will only be the beginning of a much bigger debate. As Buolamwini says, benchmarks can play their part, but more needs to be done: “Companies, researchers, and academics developing these tools have to take responsibility for placing context limitations on the systems they develop if they want to mitigate harms.”